GIS vs Geospatial Analytics: Unraveling the Differences and Synergies
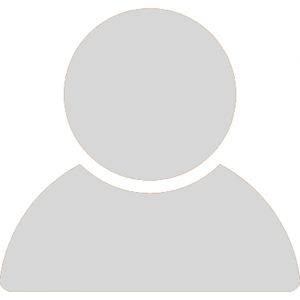
Audio : Listen to This Blog.
Introduction
Geographic information systems (GIS) and geospatial analytics are two terms widely used in the rapidly developing field of spatial data analysis and interpretation. They both deal with geographical information, but in different ways and for different purposes. In this comprehensive examination, we’ll dissect the variations, investigate the specific benefits, and learn how they supplement one another in the complex field of spatial analysis.
Understanding GIS
For many years, Geographic Information Systems (GIS) have been an important part of managing and analyzing spatial data. A GIS is basically a method for collecting, saving, organizing, and showing information about places. It provides a framework for working with location-based data by giving you the tools and options to change and display this information.
Key Components of GIS
Spatial Data: Spatial data is what makes GIS work. It includes information about the shape and location of physical objects, as well as the characteristics that go with them. The fact that this material is organized into layers makes it easy to handle.
Data Capture and Integration: GIS systems take information from many places, like GPS devices, remote tracking technologies, and polls, and put it all together on one platform.
Data Analysis: GIS uses physical research to find answers about certain places or areas. It can figure out how far something is, find the best ways, and find trends in the data.
Data Visualization: Making maps and other visuals is one of the main things that GIS does. These pictures help people understand patterns and trends in geography, which is important for making smart decisions.
Database Management: GIS handles large amounts of spatial data well, making it easy for users to search for, change, and get information.
Understanding Geospatial Analytics
Even though geospatial analytics is linked to GIS, it is a younger idea that focuses on in-depth analysis and making decisions based on data. Using powerful statistical and machine learning methods to learn more about things that happen in space is what it means.
Key Components of Geospatial Analytics
Advanced Data Analysis: Geospatial analytics goes beyond basic mapping; it involves analyzing data in depth, identifying correlations, trends, and outliers.
Predictive Modeling: This aspect of geospatial analytics uses predictive models to anticipate future events based on historical data. This makes it a valuable tool for businesses and government agencies.
Machine Learning and AI: Geospatial analytics incorporates machine learning and artificial intelligence to automate data analysis and recognize complex spatial patterns.
Real-time Data: With the rise of the Internet of Things (IoT) and sensor technologies, geospatial analytics can process real-time data, enabling instant decision-making based on dynamic information.
Differences and Overlaps
Now, let’s delve deeper into the differences and overlaps between GIS and geospatial analytics:
Focus
GIS is primarily focused on data visualization, mapping, and data storage.
Geospatial analytics concentrates on data analysis and interpretation, often involving complex statistical and machine learning methods.
Purpose
GIS is commonly used for mapping, urban planning, land management, and resource allocation.
Geospatial analytics finds applications in diverse fields such as risk assessment, market analysis, environmental modeling, and predictive modeling.
Data Volume
GIS traditionally deals with static datasets, which are vital for mapping and visualization.
Geospatial analytics handles dynamic data sources, including real-time information from IoT devices and sensors, making it ideal for forecasting and proactive decision-making.
Decision Support
GIS is invaluable for visualizing data, aiding in decision support systems, and providing an intuitive understanding of geographical data.
Geospatial analytics enhances decision-making by predicting future events, recognizing hidden patterns, and providing actionable insights based on data analysis.
Integration
Both GIS and geospatial analytics can be integrated into larger systems and workflows, depending on specific needs. They complement each other, creating a more comprehensive approach to handling spatial data.
Synergies
Although GIS and geospatial analytics have distinct roles and functions, they can work together seamlessly, offering a holistic approach to geographical data analysis. Organizations can leverage GIS for mapping and data storage, while employing geospatial analytics to extract valuable insights from that data. This synergy allows for more informed decision-making, predictive modeling, and a deeper understanding of the world around us.
Conclusion
In the ever-evolving landscape of geographical data analysis, both GIS and geospatial analytics play crucial roles. While GIS focuses on the fundamental tasks of mapping and data visualization, geospatial analytics delves deeper into data analysis, interpretation, and prediction. Understanding the differences and synergies between these two fields is essential for businesses, organizations, and professionals seeking to harness the power of spatial data. By recognizing how GIS and geospatial analytics complement each other, they can make more informed decisions, solve complex problems, and unlock new opportunities in the realm of spatial analysis.
Are you ready to leverage the full potential of GIS and geospatial analytics for your organization’s growth? Partner with MSys Technologies and discover a world of possibilities in spatial data analysis. Contact us today to explore how our expertise can help you stay ahead in the ever-evolving landscape of spatial intelligence.