5 Top AI Challenges in Cybersecurity You shouldn’t Overlook
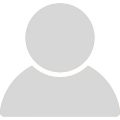
Audio : Listen to This Blog.
Advancement in technologies has created umpteen opportunities for cybercriminals to steal data. The rise in the use of cloud technology has accelerated the process of sharing of data online – information is now available irrespective of place and time. The odds are far more favorable than before for cybercriminals to get into your system.
Organizations are firefighting cyber threats at two fronts – from amateur script artists who consider hacking more as awarding than rewarding, and attacks backed by organized crime syndicate with intentions to de-stabilize operations and damage the economy. Per a report by Security Intelligence, the average cost of a data breach is $3.92 million as of 2019. Cybersecurity Ventures predicts that the damage to the world due to cybercrime will reach $6 trillion annually by 2021. This represents the greatest transfer of economic wealth in history, risks the incentives for innovation and investment, and will be more profitable than the global trade of all major illegal drugs combined. This amount will only climb up until we do away with the firefighting approach and think more proactively,
It takes a thief to catch a thief
To beat some in their own game, you must think like them. If they are fast, you must be fast; if they are cutting-edge, you must be cutting edge. To counter the threats posed by cybercriminals, organizations ought to be faster. It requires to do away with traditional security measures and embrace new age, automation-driven practices that could put us ahead of any hacker. The regular practice includes securing only mission-critical parts within an infrastructure. This leaves room open for hackers to target non-critical components. Therefore, organizations must implement comprehensive and robust cybersecurity procedures that cover every component within an infrastructure. Further, organizations should align themselves with the practice of leveraging automated scripts to facilitate continuous monitoring and reporting in real-time.
Ushering an era of proactive cybersecurity via Machine Learning
Artificial intelligence (AI) and machine learning (ML) gives an edge to modern software that are primarily created to protect from unethical cyber practices. With AI and ML, the cybersecurity software products get an extra sense to underline concurrent behavioral patterns of the workflows, assess its threat level and, based on it, alert the concerned team. The key reason why AI/Ml can perform such activity is its ability to gauge data, compare it with past actions, and derive an inference. This inference provides the security team an insight into future events that could lead to a possible cyber-attack. However, AI application is still in the nascent stages. Per IDC, one in four AI project usually ends up failing. This means there are challenges we must counter in order to make AI a success. These challenges become significant when the matter is about the organization’s data security.
Let us now analyze 5 top challenges that prevent the successful implementation of AI/Ml for cybersecurity.
1. Non-aligned internal processes
Most companies have optimized their infrastructure, especially its security components, by investing in tools and platforms. Yet, we see that they face security hurdles and fail to safeguard themselves against an external attack. This is a result of a lack of internal process improvements and cultural change that prevents capitalizing the investments in security operation centers. Further, the lack of automation and fragmented processes creates a less robust playground to defense against cybercriminals.
2. Decoupling of storage systems
Most organizations do not leverage data broker tools like RabbitQ and Kafka to initiate analytics of the data outside the system. They do not decouple storage systems and compute layers, which doesn’t allow AI scripts to execute effectively. Further, a lack of decoupling of storage systems increases the possibilities of vendor lock-ins in case of a change in the product or platform.
3. The issue of malware signature
Signatures are like fingerprints of malicious code that assist security teams in finding the malware and raising an alert. The signatures do not match the growing number of malware every year. The concern is that any change in the script of the virus makes the signature invalid. In short, signatures will only help debug malware if the code is pre-established by security teams.
4. The increasing complexity of data encryption
The rise in the use of sophisticated and advanced data encryption strategies are making it difficult to isolate an underlying threat. The most common way to monitor external traffic is via deep packet inspection (DPI) that helps filter external packets. However, these packets consist of a predefined code characteristic that can be weaponized to infiltrate in the system by the hackers. Further, the complex nature of DPI puts pressure on the firewall, slowing down the infrastructure speed.
5. Choosing the right AI use cases
More than 50 percent of the AI implementation project fails in the first go. This is because organizations try to adopt AI on a company-wide level. They often neglect the importance of baby steps – narrowing down on AI-based use cases. Thus, they miss out on initial learning curves and fail to absorb critical hiccups that often jeopardize the AI projects.
AI/ML isn’t a magic bullet rather
AI/Ml isn’t a cure-all to the activities of cybercriminals. Rather, a fierce defense that is rooted in intelligence and intuition. AI/ML will help create intelligent systems that work as a potent defensive force against activities. They could detect and alter, but they can’t reason why and how these activities were triggered. It is the security teams that need to carry out root-cause analysis of the incident/s and then remediate it.
Take Away
Mature processes, cultural alignment, and skillful teams and choosing the right AI use cases in cybersecurity are the key to the success. For this, security teams must carry out an internal audit and tick mark areas in infrastructure that are the most vulnerable. Ideally, they can start with data filtering to segregate unauthenticated sources. This isn’t the thumb rules, though. The bottom line is taking mindful steps towards adopting AI for cybersecurity.