Data Reduction: Maintaining the Performance for Modernized Cloud Storage Data
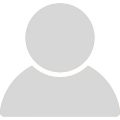
Audio : Listen to This Blog.
Going With the Winds of Time
A recent white paper by IDC claims that 95% of organizations are bound to re-strategize their data protection strategy. The new workloads due to work from home requirements, SaaS, and containerized applications call for the modernization of our data protection blueprint. Moreover, if we need to get over our anxieties of data loss, we are to really work with services like AI/ML, Data analytics, and the Internet of Things. Substandard data protection at this point is neither economical nor smart. In this context, we already talked about methods like Data Redundancy and data versioning. However, data protection modernization extends to the third time of the process, one that helps reduces the capacity required to store the data. Data reduction enhances the storage efficiency, thus improving the organizations’ capability to manage and monitor the data while reducing the storage costs substantially. It is this process that we will talk about in detail in this blog.
Expanding Possibilities With Data Reduction
Working with infrastructures like Cloud object storage, block storage, etc., have relieved the data admins and their organizations from the overhead of storage capacity and cost optimization. The organizations now show more readiness towards Disaster recovery and data retention. Therefore, it only makes sense that we magnify the supposed benefits of these infrastructures by adding Data Reduction to the mix. Data reduction helps you manage the data copies and increase the efficacy value of its analytics. The workloads for DevOps or AI are particularly data-hungry and need more optimized storage premises to work with.
In effect, data reduction can help you track the heavily shared data blocks and prioritize their caching for frequent use. Most of the vendors now notify you beforehand about the raw and effective capacities of the storage infra, where the latter is actually the capacity post data reduction. So, how do we achieve such optimization? The answer unfolds in 2 ways:
- Data Compression
- Data Deduplication
We will now look at them one by one.
Data Compression
Data doesn’t necessarily have to be stored in its original size. The basic idea behind data compression is to store a code representing the original data. This code would acquire less space but would store all the information that the original data was supposed to store. With the number of bits to represent the original data reduced, the organization can save a lot on the storage capacity required, network bandwidth, and storage cost.
Data compression uses algorithms that represent a longer sequence of data set with a sequence that’s shorter or smaller in size. Some algorithms also replace multiple unnecessary characters with a single character that uses smaller bytes and can compress the data to up to 50% of its original size.
Based on the bits lost and data compressed, the compression process is known to be of 2 types:
- Lossy Compression
- Lossless Compression
Lossy Compression
Lossy compression prioritizes compression over redundant data. Thus, it permanently eliminates some of the information held by the data. It is highly likely that a user may get all their work done without having to need the lost information, and the compression may work just fine. Compression for multimedia data sets like videos, image files, sound files, etc., are often compressed using lossy algorithms.
Lossless Compression
Lossless compression is a little more complex, as here, the algorithms are not supposed to permanently eliminate the bits. Thus, in lossless algorithms, the compression is done based on the statistical redundancy in the data. By statistical redundancy, one simply means the recurrence of certain patterns that are near impossible to avoid in real-world data. Based on the redundancy of these patterns, the lossless algorithm creates the representational coding, which is smaller in size than the original data, thus compressed.
A more sophisticated extension of lossless data compression is what inspired the idea for Data deduplication that we would study now.
Data Deduplication
Data deduplication enhances the storage capacity by using what is known as – Single Instance Storage. Essentially a specific amount of data sequence bytes (as long as 10KB) are compared against already existing data that holds such sequences. Thus, it ensures that a data sequence is not stored unless it is unique. However, this does not affect the data read, and the user applications can still retrieve the data as and when the file is written. What it actually does is avoid repeated copies and data sets over regular intervals of time. This enhances the storage capacity as well as the cost. Here’s how the whole process works:
Step 1 – The Incoming Data Stream is segmented as per a pre-decided segment window
Step 2 – Uniquely identified segments are compared against those already stored
Step 3 – In case there’s no duplication found, the data segment is stored on the disk
Step 4 – In case of a duplicate segment already existing, a reference to this existing segment is stored for future data retrievals and read. Thus, instead of storing multiple data sets, we have a single data set referred at multiple times.
Data compression and deduplication substantially reduce the storage capacity requirements allowing larger volumes of data to be stored and processed for modern day tech-innovation. Some of the noted benefits of these data reduction techniques are:
- Improving bandwidth efficiency for the cloud storage by eliminating repeated data
- Reduces storage capacity requirement concerns for data backups
- Lowered storage cost by reducing the amount of storage space to be procured
- Improves the speed for disaster recovery as reduced duplicate data makes the transfer easy
Final Thoughts
Internet of Things, AI-based automation, data analytics powered business intelligence – all of these are the modern day use cases meant to refine the human experience. The common pre-requisite for all these is a huge capacity to deal with the incoming data juggernaut. Techniques like data redundancy and versioning protect the data from performance failures due to cyberattacks and erroneous activities. On the other hand, data reduction enhances the performance of the data itself by optimizing its size and storage requirements. The modernized data requirements need modernized data protection, and data reduction happens to be an integral part of it.